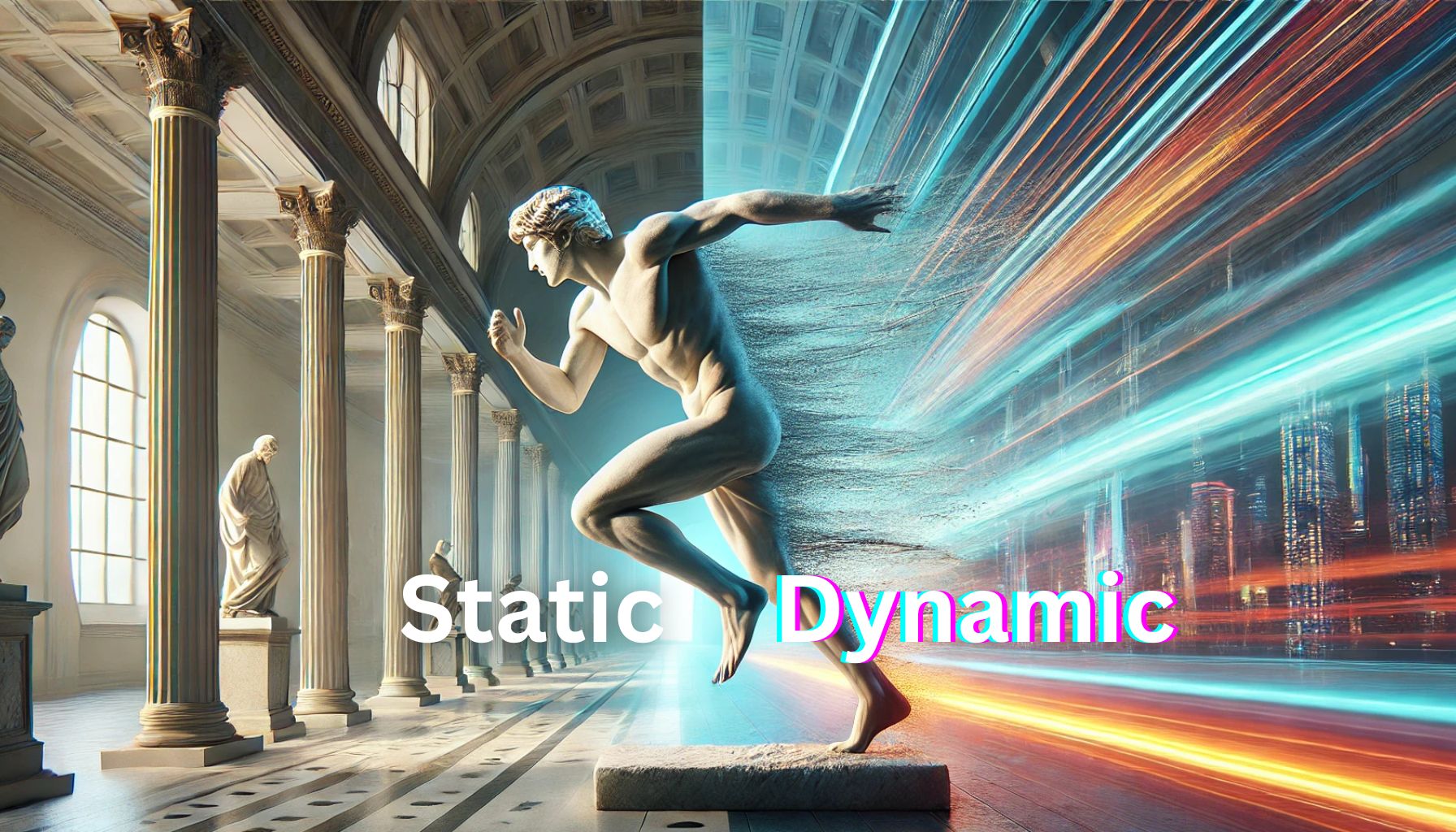
Combining Static and Dynamic Data for Holistic AI Training
Your business is drowning in data—static files gathering dust, dynamic streams flowing fast. What if you could fuse them to supercharge your AI? Here’s how to turn data chaos into a competitive edge
Static data is your business’s memory—think old invoices, customer bios, or that blog post from 2019. It’s the foundation AI leans on. Dynamic data captures real-time interactions—what customers click today, how long they linger, and what they buy now. The real-time beat keeps your AI in tune with the moment.
Fuse the two, and your AI doesn’t just work—it wows, delivering spot-on insights and game-changing moves.
The Two Faces of Data
Check out this table to learn the basic differences between static and dynamic data.
Why This Mash-Up Matters
Relying solely on static or dynamic data limits an AI model's ability to provide a comprehensive analysis. Combining the two enables:
- Contextual Understanding: Static data provides the background, while dynamic data offers the current context.
- Enhanced Personalization: AI can personalize user experiences by merging past preferences with real-time behavior.
- Improved Predictive Power: Models trained on a mix of historical patterns and live updates can predict future outcomes more accurately.
Steps to Integrate Static and Dynamic Data for AI Training
Here are the steps to integrate static and dynamic data for AI training.
- Data Collection and Consolidation
- Aggregate static content from existing databases and content repositories.
- Wire up systems to capture real-time user interactions and system events.
- Data Preprocessing
- Clean and standardize both data types to ensure consistency.
- Handle missing values and outliers appropriately.
- Feature Engineering
- Extract meaningful features from static data (e.g., user preferences from past behavior).
- Generate real-time features (e.g., most recent activity, time since last interaction).
- Model Training and Validation
- Use machine learning algorithms that handle mixed data inputs, such as deep learning models or ensemble methods.
- Validate models on both historical and live datasets to ensure robustness.
- Continuous Monitoring and Updates
- Regularly update static data sources to reflect changes in business priorities.
- Implement real-time data pipelines for continuous model improvement.
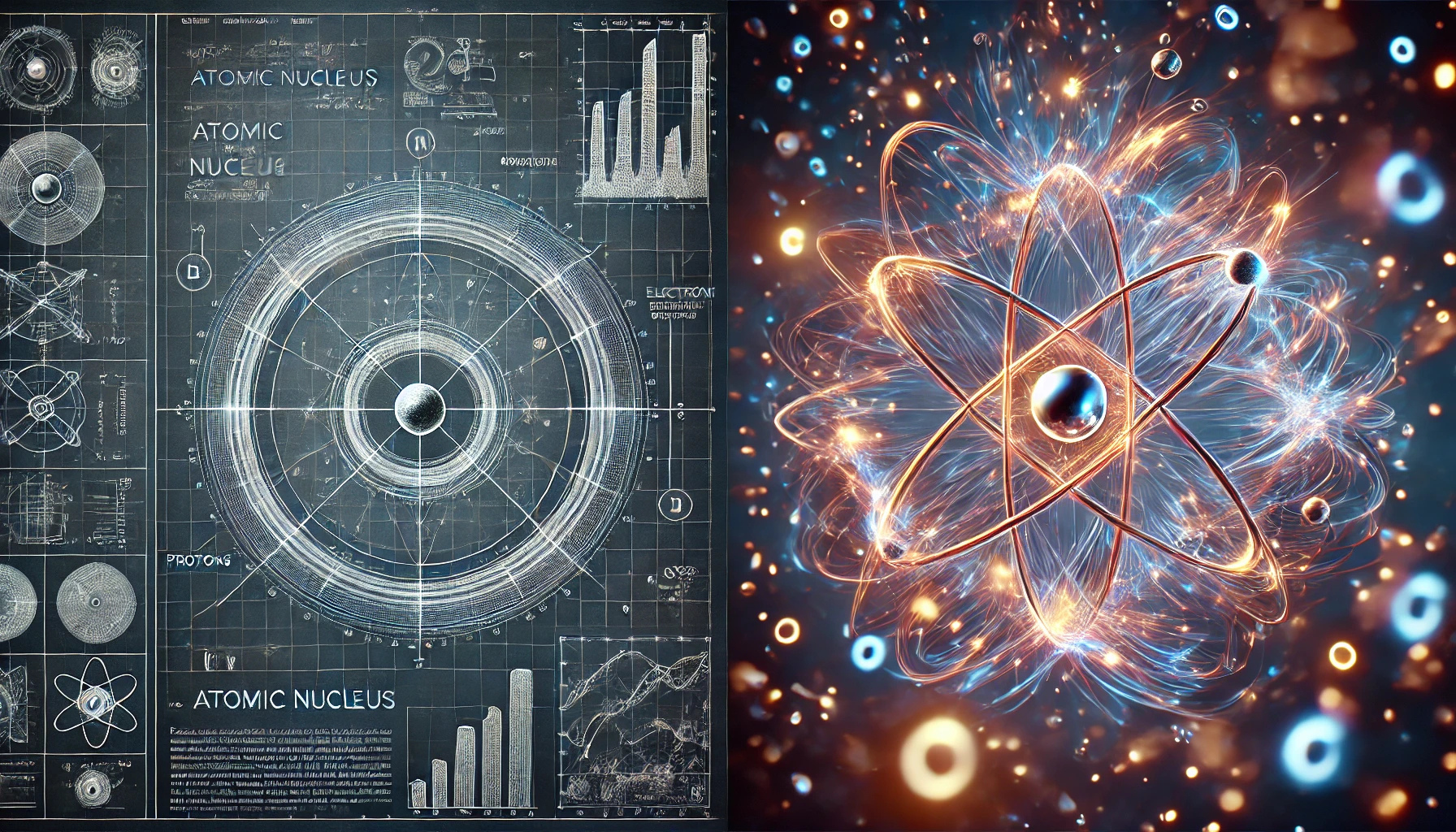
Conclusion
Master this data dance to combine data science and Big data. Your AI doesn’t just analyze—it anticipates, delights, and dominates. Your rivals? Still stuck in yesterday’s data swamp!
Businesses that master this integration will unlock deeper insights, deliver better customer experiences, and stay ahead of their competition. As AI technology advances, the ability to harness all available data streams will become the cornerstone of innovation and growth.
Related Post
RECOMMENDED POSTS
RECOMMENDED TOPICS
TAGS
- ai
- artificial intelligence
- machine learning
- deepseek
- agentic ai
- ai development
- openai
- llm
- open source
- ai/ml
- artificial intelligene
- data visualization
- bpa
- automation
- healthcare
- modern medicine
- growth engineering
- growth hacks
- data roles
- data analyst
- data scientist
- data engineer
- qwenlm
- xai
- ai model
- chatgpt
- data science
- dynamic data
- static data
- empathy
- it development
- leadership
- gpt
- llm models
- climate change
- test
ABOUT
Stay ahead in the world of technology with Iowa4Tech.com! Explore the latest trends in AI, software development, cybersecurity, and emerging tech, along with expert insights and industry updates.
Comments(0)
Leave a Reply
Your email address will not be published. Required fields are marked *